Artificial intelligence is, without a doubt, increasing its prevalence in radiology circles. As it takes an average of 17 years to fully adopt a new medical technology into an industry, AI in radiology is arguably still in its infancy[1].
June 29, 2022 – The vast majority of new AI products for radiologists have only reached the market in the last few years[2], but have already seen high success rates in improving diagnostic accuracy.
A deep learning-based AI tool is valuable in any radiologist’s toolbox in any radiologist’s toolbox, and understanding its use, developments, and limitations – if not already essential – will rapidly become key to maintaining a practitioner’s relevancy in radiological practice.
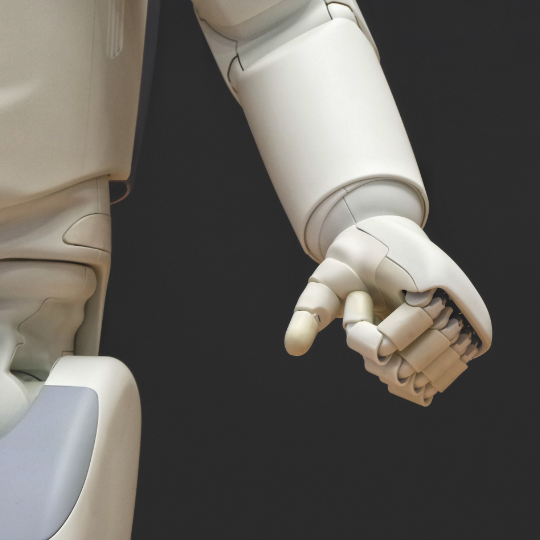
How AI systems in radiology work
Deep learning-based AI tools are trained on data from real cases. In most current training systems, a radiologist informs the AI as to which images include clinical abnormalities and which represent healthy cases. By comparing data and visual similarities, the algorithm learns to recognise physical patterns which may constitute disease presences.
Many AIs learn to recognise these dangerous patterns on a very small scale, detecting incredibly small tumours that range only 1-3 millimetres in size. Such small lesions may show up as only a few pixels on a diagnostic scan, making it unlikely that a radiologist could detect them with the naked eye.
Will radiologists become defunct?
Although human vision can greatly limit diagnostic capabilities, professional radiologists should not worry about being replaced by machines in the near future. Deep learning-based AIs can identify potential concerns and flag them to radiologists, but the tasks of confirming a diagnosis, determining a tumour’s biological aggression, and devising suitable action must still fall to a human.
In the words of computer vision specialist Ulas Bagci, ‘computers are good at very local tasks. Humans are much better at global tasks.’ While AI systems can spot potential concerns in an image, they still need human input to render a definitive diagnosis by combining multiple data sources (such as blood tests and physical exams)[3].
Furthermore, deep learning-based AI systems learn to recognise patterns upon which they have been trained. As a result, they can accurately spot common problematic lesions when they are smaller than an average radiologist may notice, but are less successful at recognising uncommon and unusual presentations which do not match their training. The radiologist’s eye remains essential to recognising difficult cases, supplemented by AI systems that help spot smaller concerns and let professionals focus on more nonstandard ones. Such collaborative approaches between AI and radiologists in the radiological workflow are referred as 'augmented intelligence'.
AI has not replaced the radiologist, and it is not likely to do so any time soon. But as Dr. Curtis Langlotz said in 2018, ‘AI won’t replace radiologists, but radiologists who use AI will replace radiologists who don’t’[4]. Learning to leverage this ground-breaking technology is key to maintaining relevance in the future.
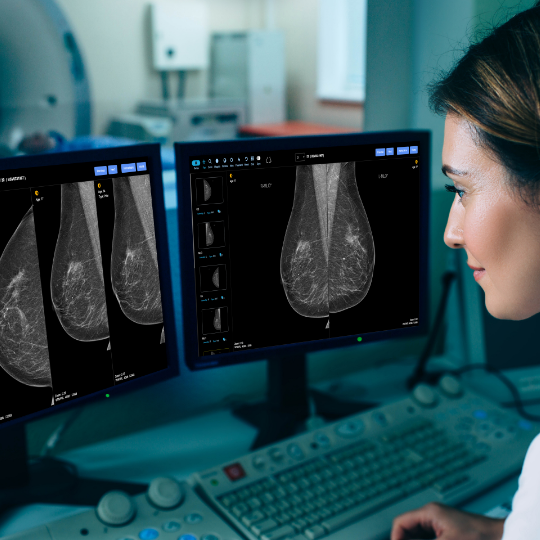
Barriers to widespread implementation
Perhaps one of the greatest difficulties in implementing this potentially life-saving technology is a lack of education. Learning institutes are gradually adding AI training and discussion to their radiological curricula, though it is not being adopted as rapidly as it is developing.
At current, most AI training programs for radiologists are short, stand-alone sessions that are offered passively. With little accompanying hands-on training to solidify learning , they risk being ineffectively implemented or forgotten quickly.
Academic institutions such as universities are – surprisingly – minimally involved in AI training, resulting in most training programs being provided by professional institutions and commercial companies. Many of these businesses have a financial stake in creating or advertising specific artificial intelligence systems, potentially resulting in biased learning that primarily promotes a single product.
Though expectations may change in the coming years, the task of understanding and training to use practical AI use still falls to the individual radiologist.
Learn more about AI in radiology
Lifesaving technology and education on how to use it effectively deserves to be widely accessible and broadly implemented. In keeping with our mission to improve diagnostic accuracy on a global scale, DetectedX proudly hosts a number of lectures on understanding AI and implementing it in diagnostic imaging.
Presented by AI specialist and data scientist Dr Ziba Gandomkar, these lectures create a valuable, actionable launching point for understanding and implementing artificial intelligence in diagnostic imaging (and provide over an hour of CME and CPD accredited training). To begin your free trial of DetectedX’s radiology online learning platform, visit our online learning centre or contact our team to discuss corporate options.
Dr Ziba's AI learning principles lectures is available to paid users of DetectedX. Click here to login or signup here.Â
About DetectedX
DetectedX’s Radiology Online Learning Center, focusing on diagnostic accuracy and driven by artificial intelligence, is revolutionizing disease detection in 150 countries. The on-demand, web-based training platform has been proven to improve the accuracy of diagnosing difficult cases by 34%. DetectedX was founded by Professor Patrick Brennan, a leading medical radiation scientist with $40+M in research funding and 500+ publications, Prof. Mary Rickard, Australia’s number one breast imaging clinician expert scientist who pioneered Australia’s Breast Screening program, and Dr. Moe Suleiman, one of the world’s leaders on optimizing radiologic interpretation.